Ph.D. Candidate
Email: zheliu1@seas.upenn.edu
Phone: (404) 472-6712
220 S 33rd St
Towne 347
Philadelphia, PA, 19104
Bio
2018: Master of Science in Chemical Engineering, Georgia Institute of Technology
2016: Bachelor of Science in Chemical and Biomolecular Engineering, Biotechnology option, Georgia Institute of Technology
Current Research
My research investigates programming and patterning the active material. The active material such as liquid crystal elastomer has a unique property of response to a specific stimulus, like heat or light, which results in it being an excellent candidate as an actuator. To design an actuator requires a sophisticated methodology to program the active material. The first part of the research is to fabricate a thermal oscillator by using the liquid crystal elastomer under a constant heat flux. The second part of my study is to pattern the active material by using the magnetic field to generate non-trivial actuation.
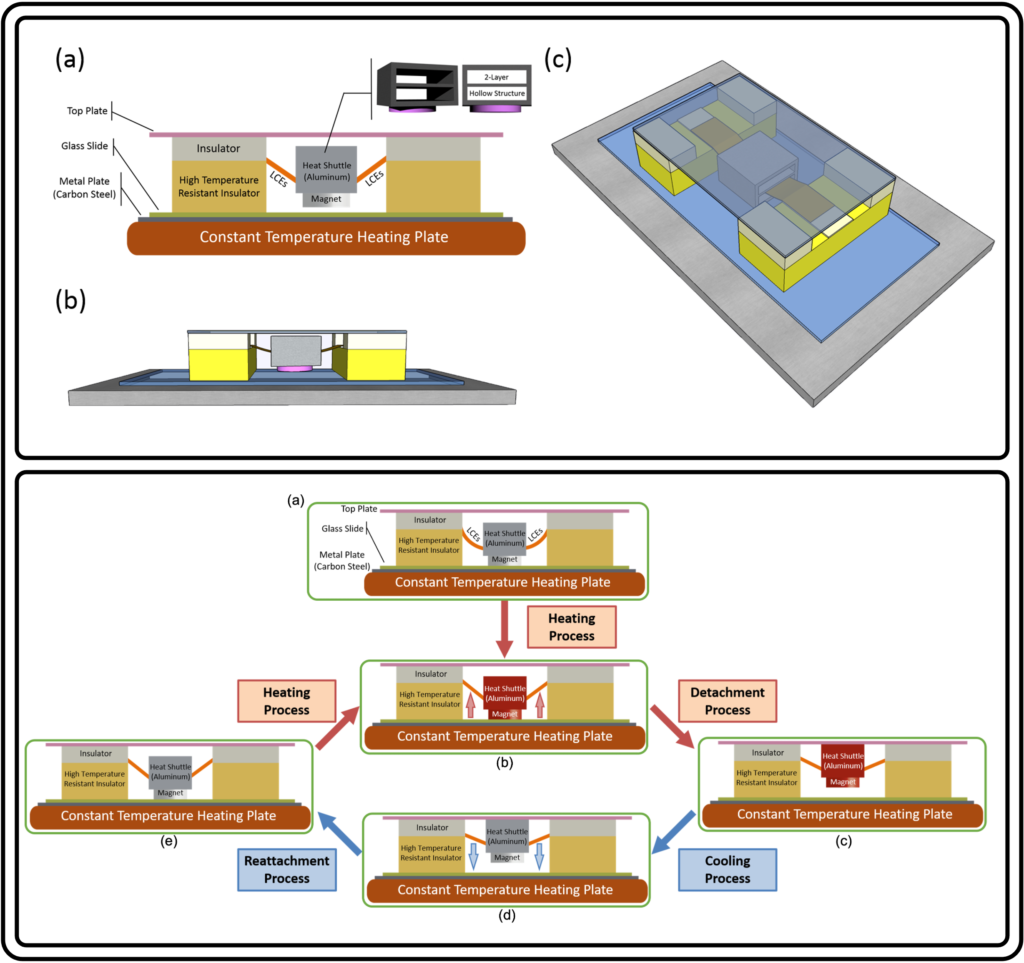
Previous Research
During my undergraduate at Georgia Tech, I first joined Dr. Sven Behrens’ research lab and worked with Ph.D. student Abiola. The research investigated how particles stabilize droplets in the three-phase system. He and I utilized the surface-modified silica particles to change the equilibrium state of immiscible liquid droplet structures. Also, we designed a novel microcapsule for an aqueous herbicide to improve its biological efficacy.
After that, I started working with Ph.D. student Aditi in Dr. Andrew Bommarius’ research lab. She and I tried to optimize the Amyloid Beta1-24 Production in the lab-scale. The research identified the candidates of competent cells that have high expression of Amyloid Beta. We also develop a method to keep protein from aggregation and to obtain a monomeric solution.
In my graduate education, I studied optimization in Dr. Fani Boukouvala’s research group. I attempted to improve models of crystallization by using historical experimental data. The approach is to generate a data-driven model with the help of machine learning technique and couple the model with mechanistic models to get a better prediction.